Tianyu Chen
University of Texas at Austin; tianyuchen@utexas.edu
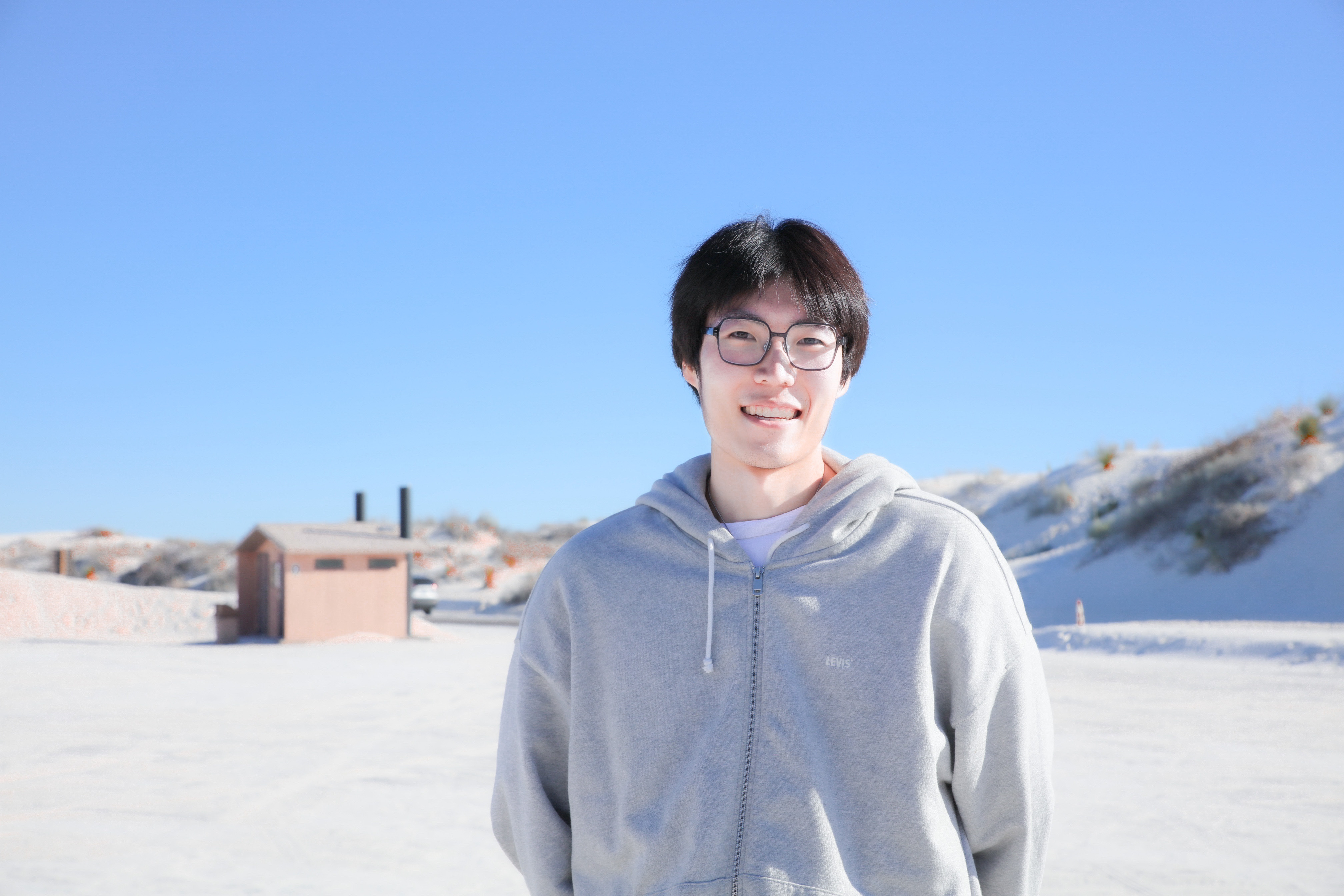
White Sands, New Mexico
I am a second-year PhD student at the University of Texas at Austin, supervised by Professor Mingyuan Zhou. Before joining UT, I obtained my master’s degree in Statistics from the University of Chicago, where I closely collaborated with Kevin Bello, Bryon Aragam, Pradeep Ravikumar, Francesco Locatello, and supervised by Professor Jingshu Wang. I earned my Bachelor’s degree in Statistics from Fudan University, where I spent some of the most memorable moments of my life.
Research Interests: I have broad research interests in statistical machine learning. Specifically, my research interests lie in:
- Generative Models: e.g., diffusion models, distillation, VAEs, and inverse problems.
- Reinforcement Learning for LLM: e.g. offline RL, online RL for training LLM.
- Causal Inference: e.g., graphical probabilistic models, causal representation learning.
- Statistical Sampling: e.g., neural posterior sampling.
- Bioinformatics: e.g., single-cell data and multi-omic data integration.
I am open to Summer Internship 2025 opportunities. ☀️ Please don’t hesitate to contact me if you see a good fit or have an opportunity for me. 📧
news
Mar 12, 2025 | Our new paper, Denoising Score Distillation: From Noisy Diffusion Pretraining to One-Step High-Quality Generation, introduces a novel approach to training a one-step image generator using only noisy images. Remarkably, our method achieves FID scores comparable to diffusion models trained on clean images. Beyond proposing a new solution to inverse problems through distillation, our work demonstrates that distillation is not merely an acceleration technique but also enhances generation quality compared to the teacher diffusion model—both empirically and theoretically. |
---|---|
Feb 01, 2025 | Our new paper Conditional diffusions for amortized neural posterior estimation in using diffusion model to do amortized simulation based inference is accepted by AISTATS 2025. |
Oct 01, 2024 | Our new papers Diffusion Policies Creating a Trust Region for Offline Reinforcement Learning and Identifying General Mechanism Shifts in Linear Causal Representations are accepted by NeurIPS 2024. See you in Vancouver! ![]() |
selected publications
- PreprintDenoising Score Distillation: From Noisy Diffusion Pretraining to One-Step High-Quality GenerationPreprint., 2025